Healthcare Chatbot Design Toolkit
Click Here for ChatPal Toolkit PDF
Different language versions of toolkit
- Ohjekirja terveyteen liittyvän chatbotin suunnitteluun (Finnish toolkit)5.05 M
- Verktyg för samskapande av chattbotar som ska användas i hälso- och sjukvården (Swedish toolkit)5.04 M
Authors: Courtney Potts1, Raymond Bond1, Maurice Mulvenna1, Michael McTear1, Fred Booth1, Frida Lindström2, Catrine Kostenius2, Indika Dhanapala3, Alex Vakaloudis3, Brian Cahill3, Kyle Boyd4, Thomas Broderick5, Andrea Bickerdike5, Con Burns5, Edward Coughlan5, Heidi Nieminen6, Anna-Kaisa Vartiainen6, Lauri Kuosmanen6.
Affiliations: 1 Ulster University, School of Computing, Belfast, United Kingdom 2 Luleå University of Technology, Department of Health Sciences, Luleå, Sweden 3 Munster Technological University, Nimbus Research Centre, Cork, Ireland 4 Ulster University, School of Art, Belfast, United Kingdom 5 Munster Technological University, Department of Sport, Leisure and Childhood Studies, Cork, Ireland 6 University of Eastern Finland, Department of Nursing Science, Kuopio, Finland
Citation: Potts, C., Bond, RR., Mulvenna, M., McTear, M., Booth, F., Lindström, F., Kostenius, C., Dhanapala, I. S. A., Vakaloudis, A., Cahill, B., Boyd, K., Broderick, T., Bickerdike, A., Burns, C., Coughlan, E., Nieminen, H., Vartiainen, A-K., & Kuosmanen, L. (2022). Toolkit for the co-creation of health-based chatbots. (1 ed.) Ulster University. doi.org/10.21251/g9jd-5067
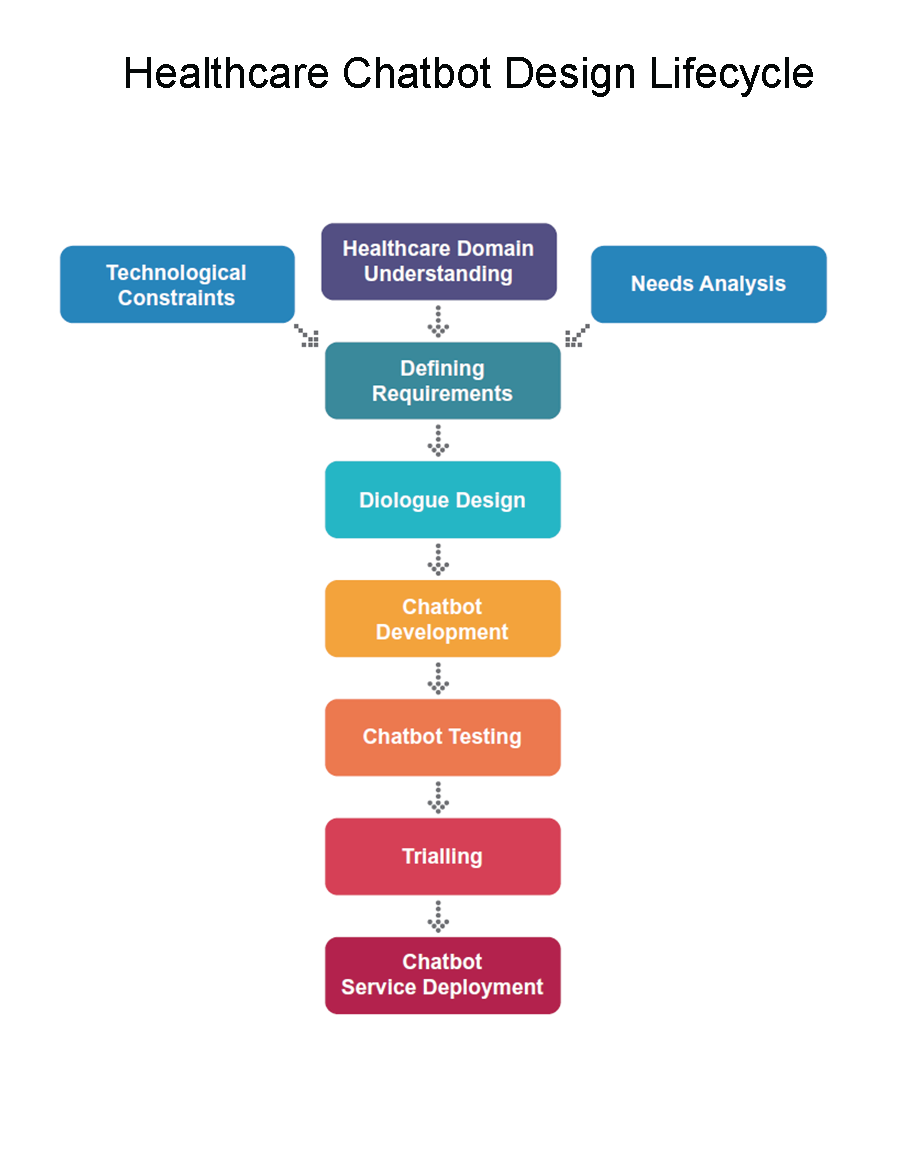
Healthcare Chatbot Design Lifecycle
This toolkit provides guidelines for designing and developing a healthcare chatbot. In this toolkit you will find recommended activities and methods which were used to adopt a stakeholder-centred approach, which is important for responsible design of digital health technologies. For each section below, you can find further helpful information, suggested steps to follow, and expected outputs should you follow our recommendations. These recommendations are based on our experiences throughout the ChatPal project. You can find out more about the project here: https://www.youtube.com/channel/UCeYBbGKE0CGx08L311YZgRw
Stakeholder Centered Design
It is crucial to look at approaches for responsible healthcare chatbot design which could consider three things 1) what users say they need, 2) what chatbots and features mental health professionals would endorse, and 3) what AI chatbots can do well. Chatbots can easily handle scripted dialogues with predefined replies or limited free text responses, and if users wanted a chatbot to self-diagnose or screen then it could be used to collect symptoms and use a decision flow to suggest a diagnosis. However, professionals may not be in support of this as it may result in a false diagnosis, which could limit its credibility and widespread adoption. Alternatively, chatbots could be used for answering questions and signposting to paid mental health services, however, users may not want this type of application to direct to paid services and thus may avoid the technology altogether. Another example is a chatbot that supports free text, attempting to detect when a user is feeling depressed and tries to respond in a way that improves the person's mood. This may be endorsed by professionals but given the limitations of AI the responses may be inappropriate if the chatbot failed to understand what the user said or if it gave inappropriate advice. Therefore, a successful digital intervention could be thought of as the intersection between what users want and say they need, what professionals advocate and what AI does well. We propose activities and methods which strike a balance between these three things.
Approaches for responsible healthcare chatbot design could consider three things
1) what users say they need, 2) what chatbots and features mental health professionals would endorse, and 3) what AI chatbots can do well.
We propose activities and methods which strike a balance between these three things:
1. What artificial intelligence (AI) can do well
2. What people want and or need
3. What professionals will endorse
Therefore, a successful digital intervention could be thought of as the intersection between what users want and say they need, what professionals advocate and what AI does well.
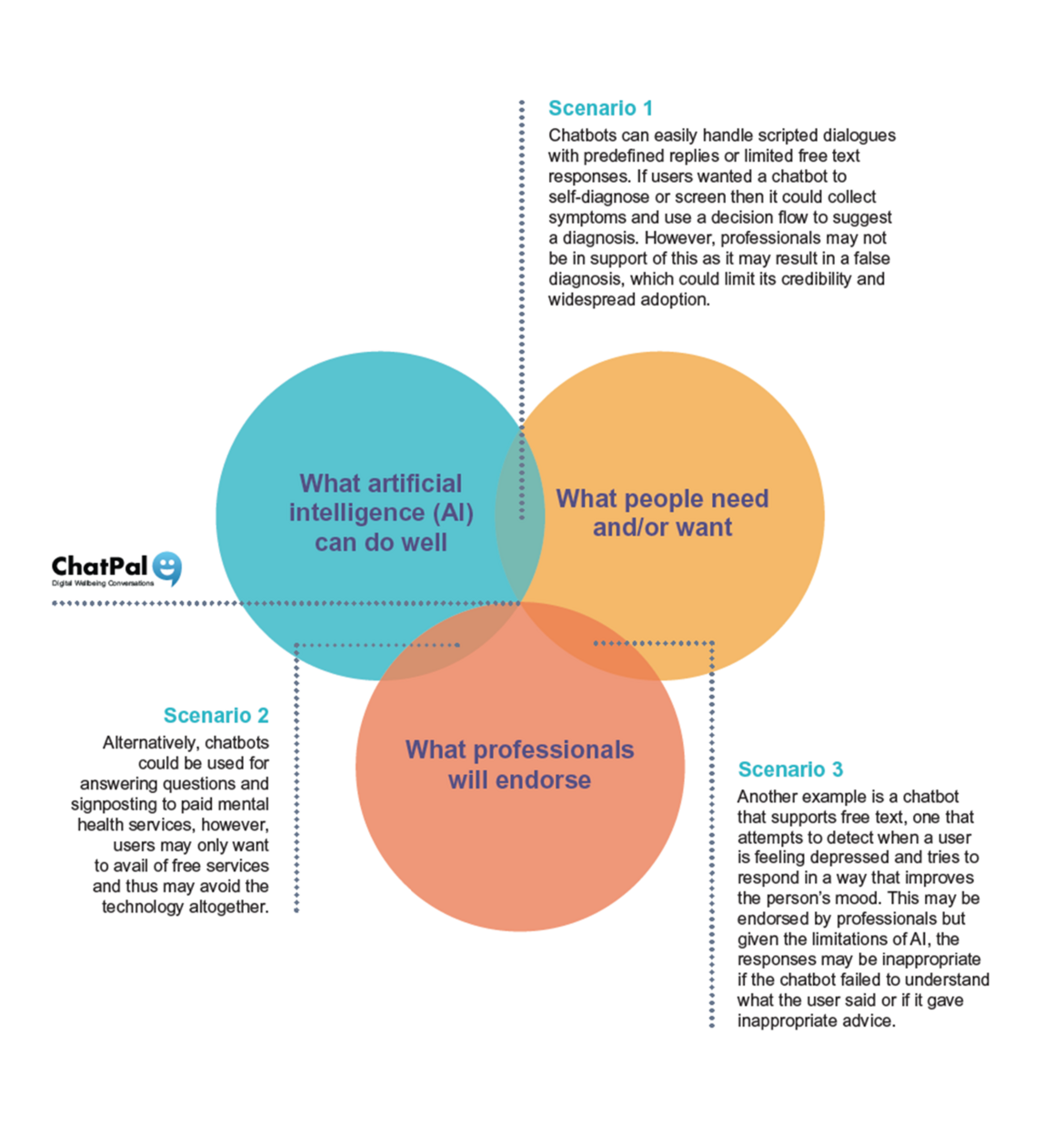
Healthcare Domain Understanding
The first step in healthcare chatbot design planning should always be to understand the domain. Undertaking a literature review in the relevant area can be used to identify what has already been done and what gaps exist.
At this stage it is useful for technologists to converse with healthcare professionals, firstly to find out what health services are currently available. This also allows healthcare professionals to share domain knowledge with technologists, and healthcare professionals can understand how technology could be used in their domain. This is crucial in the co-production process, as technology design should be domain led. Surveying health professionals to gather opinions on what they would like to see in a chatbot or how they see chatbots complementing health services can be useful at this stage. These activities provide a good starting point to inform chatbot design as you can determine the purpose of the chatbot.
Our Recommendations
- Carry out a literature review
- What has already been done in this area?
- What gaps exist in the current literature?
- Round table discussions/ workshops with technologists and healthcare professionals to share knowledge
- Assess attitudes, preferences and endorsements from healthcare professionals
- E.g. using survey (Sweeney et al. 2021)
- Establish chatbot purpose
- What will the chatbot be used for?
- Who are the end users?
- What languages will the chatbot be available in?
Expected output(s): literature review, opinions from healthcare professionals on what digital health technologies they would endorse
Technological Constraints
The next step is to identify the technologies to be used.
This includes the platform (e.g. mobile, web application etc), modality (written text or voice) and framework which will be used to build the chatbot. Many great open source frameworks for chatbot development exist, but the best option will depend on the features or scenarios the healthcare chatbot will address.
To help decide on the technology to proceed with, it is useful to consult an expert in the field. It’s important to consider the pros and cons of artificial intelligence (AI) and natural language processing (NLP). There are two main issues in NLP: Natural Language Understanding (NLU), which is concerned with understanding the user’s input, and Response Generation, which involves providing an appropriate response.
Depending on the application, limiting the amount of free text user input and allowing users to select from predefined responses can help to alleviate the problems associated with NLP. However the downside of this is that it’s not possible to elicit input from the user, other than selecting from pre-programmed replies.
One way around this would be to allow free text but attempt to constrain the dialogue with carefully designed prompts. It is a difficult issue and in safety critical domains it is better to err on the side of caution. It is also useful to plan how the chatbot would respond to users should they say something unexpected.
This is particularly important in the area of mental health, for example if someone should disclose that they are at risk of harming themselves then the chatbot should have a suitable response, such as encouraging the user to seek external help by signposting to crisis helplines. For example, this has been documented previously in 2018, when popular mental health chatbots were unable to appropriately deal with reports of child sex abuse (https://www.bbc.co.uk/news/technology-46507900)
Keyword triggers could be utilised here to direct users to appropriate advice and support.
Another study by Bickmore and colleagues reported on instances where Siri, Alexa and Google Assistant gave poor health advice which could have resulted in some degree of patient harm, even including suggestions that could have potentially been fatal (Bickmore et al. 2018). It is important to consider the ethical issues with human-computer conversations and be transparent with users, so they do not ‘overtrust’ in the information given by the chatbot, and signpost to external help or resources to avoid unintended consequences.
●Identify strengths and limitations to inform the choice of technology
● Focus on what the technology can do well and avoid areas of weakness (especially if the chatbot is delivering health related advice)
● If zero cost is an option, then chose an open source chatbot development framework
● Consider choosing a chatbot development environment where access to the data is private and standalone (e.g. ensure third parties such as social media platforms do not have peoples health data when they interact with the chatbot. If this is not possible ensure that this is disclosed to users and they consent to which organisations will have their data)
● Consider implementing keyword triggers and plan for fallback messages/ chatbot responses for unexpected inputs and edge cases Expected output(s): final decision on device, modality, and platform; risk assessment plan to address potential ethical concerns
Expected output(s): final decision on device, modality, and platform; risk assessment plan to address potential ethical concerns.
Needs Analysis
It is important to better understand the digital health needs and requirements amongst the intended user groups for the chatbot.
The first step is identification of relevant stakeholders, as this is an integral part of the stakeholder-centred design process. Needs analysis workshops or surveys with stakeholders and target users can be utilised at this stage (Potts et al. 2021).
Our Recommendations
- Identify stakeholders
- Host workshops targeted at user groups & stakeholders to identify user needs. E.g. Participants
- Invite end users or groups with similar demographic as expected end users & stakeholders Activities
- Discussion around the healthcare needs of end users
- Demonstration of similar digital health technologies
- Development of chatbot persona (gender, age, personality and character traits)
- Co-writing conversational dialogues they would like to see in a chatbot and/ or questions they would like the chatbot to ask
- Collection of user stories (“As a < type of user >, I want < some goal > because < some reason >”)
- Alternatively, surveying user groups & stakeholders is another approach which could be used to gather user needs
Expected output(s): user needs and requirements.
Defining Requirements
Once the stakeholder centred design activities have been completed, the next step is to define the chatbot requirements.
The requirements can be derived from the gathered knowledge of what technology can do well, what professionals endorse and what people need or want. Initially, this may be a long list of requirements that can then be refined for the final features.
Our recommendations
● Adopt a co-production approach to generate solutions which address user needs
○ E.g. Multidisciplinary design thinking workshops with health professionals and technology experts. If hosting online workshops, then virtual whiteboards can be used to facilitate co-production where attendees can submit their ideas, anonymously (allowing for honest opinions and a more democratic data collection exercise)
● Generate a list of requirements from stakeholder-centred design activities
● Prioritise features from the list of requirements
○ E.g. Using workshops to utilise independent voting and ranking of requirements. Individuals from multidisciplinary teams can anonymously rank features based on perceived importance and how well they fit user stories
● Decide on final chatbot features based on prioritised requirements. Thematic analysis can be used to classify the final features into different higher level thematic groups (each group being a cluster of related features/requirements). Expected output(s): list of final healthcare chatbot features.
Expected output(s): list of final healthcare chatbot features
Dialogue Design
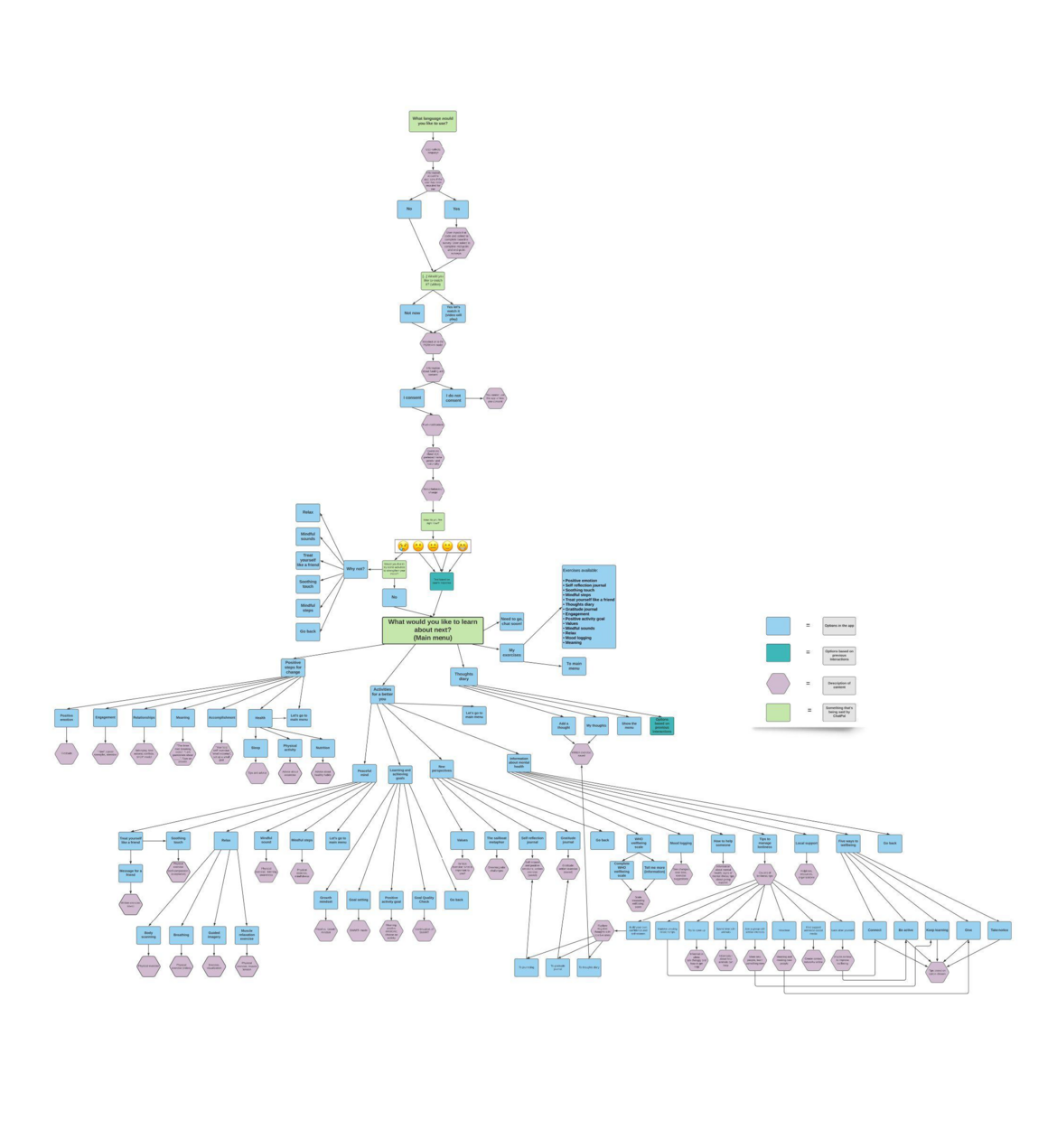
Once the final features have been decided, the next step is finalising plans for designing and writing the dialogues (chatbot content design).
The style of interactions should be considered, including the type (pre-defined, controlled, open) and control (system controlled, user controlled, both can control). If the healthcare chatbot is text based, then the addition of multimedia (images, videos, gifs) could be used to improve the user experience (UX).
Personalisation can also improve the UX so it is important to decide if and how the chatbot will use personal information about the user (name, age, gender, previous interactions etc). Chatbots can also make use of external knowledge, such as database records or online health information if necessary. It is beneficial to work in a multidisciplinary team for dialogue content development, including those with expertise in conversational UX design, as well as healthcare professionals working directly with patients or clients and healthcare professionals in academia.
Healthcare domain experts are the best people for writing the initial content for the chatbot dialogues, but it is important that they follow good design principles (Cameron et al. 2018b). It is vital to inform users of the limitations of chatbot technology upfront (at the very beginning of the conversation), for example making them aware that chatbots only have limited intelligence. When writing content to meet the requirements, keep the desired chatbot persona in mind to ensure consistency. It’s also important to think about how user inputs are used. For example, if a user discloses information about an event and the chatbot reiterates this back to the user many weeks later - then the user might feel a sense of erriness as they might have forgotten that they disclosed this information and/or they feel under surveillance as a result.
Once the scripts have been written then you can evaluate and sign off on final content. For this it may be useful to have a series of questions or criteria to quality assess each of the dialogue scripts. See Deibel et al 2021 for further reading on Conversational UX design. If the chatbot is multilingual then this is a good point to begin translating content, once the scripts have been finalised.
A good way to visualise all of the content in the app is to create a flow diagram or chart showing the features and where to access them. This can be shared with end users or healthcare professionals so they can clearly see the options available and can discuss these with their clients.
The flow diagram created for the ChatPal app can be found below as an example. This kind of flow diagram can also help the design team to identify dead ends in the chatbot experience (e.g. is there a response if a user selects ‘No’ via a quick reply button or did the designers accidently ignore this dialogue pathway).
A PDF version of this diagram can be downloaded from https://chatpal.interreg-npa.eu/resources/
Our Recommendations
● Finalise design decisions for the healthcare chatbot in terms of modality and use of multimedia or voice; style of interaction; personalisation; and external knowledge.
● Carry out multidisciplinary content development and script design activities led or informed by conversational design experts and best practices
○ E.g. workshops where dialogues are co-designed as a group using collaborative tools such as Google documents
● Be transparent and inform users of technology limitations upfront at the onboarding phase of the chatbot
● Consider how user data is used by the chatbot and whether there is likely to be confusion or an ‘uncanny valley’ (Mori et al. 2012) - feeling uneasy towards artificial representations of humans such as chatbots
● Translate final content into other languages if applicable
● Apply criteria for assessing and signing off scripts. The following questions are derived from two existing app assessment tools (Enlight - Quality assessment section and user version of Mobile App Rating Scale uMARS) and one “best practices” publication (Cameron et al. 2018a). The question on the chatbot personality will be specific, depending on the intended use of the bot and desired persona from user groups. Questions are modified to assess a single chatbot conversation and address the conversation flow, quality of information, cultural suitability and the chatbot persona.
1. Conversation flow
● Is there a suitable amount of variance in content (emojis, gifs etc)? (Cameron et al. 2018a)
● Is there a suitable amount of conversational elements emulating real conversation (reciprocity, conversational delights like the use of humour/jokes etc)? (Cameron et al. 2018a)
● Does the chatbot use appropriate language and wording for the targeted use of the bot? (Cameron et al. 2018a)
2. Quality of the information
● Is the information provided accurate? Are there evidence-based techniques relevant for achieving the desired aim of the program? (Baumel et al. 2016)
● Is the information provided in a clear and appropriate way for the target audience? (Baumel et al. 2016)
● Is there sufficient information in this conversation without any omissions, over-explanations, or irrelevant data? (Baumel et al. 2016)
● Is the content of this conversation correct, well written, and relevant to the goal/ topic of the healthcare chatbot? (Stoyanov et al. 2016)
3. Cultural aspects
● Do you perceive this conversation as culturally suitable? (Cameron et al. 2018a)
4. Persona
Based on the conversation draft, does the wording fit with the desired:
● Age of the chatbot?
● Gender of the chatbot?
● Chatbot persona in terms of personality traits?
Expected output(s): final chatbot content
Chatbot Development
Chatbot development can begin once the content has been finalised.
This stage involves the development and release of a minimum viable prototype and we recommend taking an agile approach for this. When developing the chatbot, it is a good idea to incorporate event logging, where each interaction is recorded in the chatbot log database for future analysis. This will allow you to explore engagement and which features people use most often for example.
Consideration should be given to allow the chatbot to support multimedia content where possible, and incorporating app notifications to encourage usage of the healthcare chatbot. Including ecological momentary assessment (EMA), which are questions presented and answered by users ‘in the moment’ may also be beneficial.
Depending on the healthcare application, EMA can be used for a variety of purposes such as tracking mood across hours of the day (Bond et al. 2019). Another example from Goldstein and colleagues includes a mobile app that they developed to help prevent dietary relapses in overweight individuals by asking participants to record slip ups in their diet along with a range of potential triggers using EMA (Goldstein et al. 2017). Dynamic computational modelling was used to estimate the level of upcoming risk for lapse of eating behaviour and interventions including text messages were delivered to help prevent lapses (Goldstein et al. 2017).
Our recommendations
● Record user event log data automatically so it can be analysed
● Embedding EMA within the chatbot can be helpful (e.g. Questions such as what is your current mood?)
● Consider the ability of the chatbot to support multimedia and notifications to keep users engaged and improve user retention.
Expected output(s): chatbot prototype.
To hear about the theory behind chatbots, how they work and how we have created ChatPal, visit this page https://www.youtube.com/watch?v=GnPChzAw0CI - Video where Dr Alex Vakaloudis (Munster Technological University) is interviewed about chatbot development.
Chatbot Testing
Following development of the initial prototype, testing can be carried out.
For this, it is useful to start off by working in a multidisciplinary team to carry out system testing for errors, which can be reported to the developers and fixed. Once the initial issues have been resolved, the healthcare chatbot is ready for further testing with users.
Usability testing with a small number of participants can be helpful to obtain feedback to optimise the user experience and evaluate the chatbot early on, as well as throughout the development process.
Once technical errors have been resolved, the healthcare chatbot can be trialled with the desired population.
Our recommendations
- Multidisciplinary testing and bug logging
● Usability testing (n=~10-20 participants) with prototype and subsequent versions of the app
● Gathering iterative feedback from users can be used to inform for further development and future versions of the app.
Expected output(s): optimised chatbot prototype
Trialling
A good way to measure real world engagement is to test the app by trialling it ‘in the wild’. In addition, it is useful to have a researcher provoked trial such as pilot, feasibility, or randomised controlled trial with end users. In this toolkit, we refer to ‘in the wild’ trials as cases where the chatbot is organically downloaded from app stores and used in the real world.
In the wild users give consent remotely to allow researchers to analyse the chatbot log data and any other interaction data collected through the app. In these cases, the data collected is more naturally occurring but perhaps lacks the rigour of a more controlled study.
In contrast, we see a researcher provoked trial as one that is more controlled in terms of recruitment and data collection, and the participants would perhaps have more contact with the researcher. Based on our experiences we recommend carrying out a short trial first to identify and resolve technical or logistic issues and problems with data collection.
We opted for a short 4-week trial initially and then fine tuned the protocol ahead of a longer 12-week trial. In these trials, questionnaires and scales can be used to cross examine or measure different outcomes at regular intervals throughout the trial period. When selecting outcome measures, think about the cause and effect of the digital solution, and you may even want to measure multiple different outcomes.
Consider utilising different approaches to gather feedback. For example, prompting the user to give feedback in the app itself or completing external surveys, following up with participants via telephone, email or in person and focus groups to gather vital feedback on user experience. On the following pages you can find the trial design that was used for trialling the ChatPal app.
Informed consent should be obtained from participants who are enrolling on the trial. The simplest way to do this is to incorporate digital consent into the app, with short concise statements stating what the trial involves and how their data will be used. Participants should be able to “opt-in” to consent to the storage and analysis of their data, as this is good ethical practice.
In terms of trial recruitment, volunteers can be sought through organisations for example universities, mental health or other health services and third sector organisations. Snowballing approaches are useful to encourage others to join the trial, as participants can share details with friends, family and colleagues. Publicising details online in news articles and advertising on social media can encourage participation and traditional marketing approaches, such as putting up posters in local shops and community centres is a useful way to target non-digital natives.
Our recommendation for an approach that works well is to engage with participants in person to invite them to take part in trials, and follow up in person where possible. This is likely to encourage people to keep going in the trials, and can be useful to gather feedback on the product. Throughout the trials, ensure that participants have adequate support and access to healthcare professionals should any issues arise. Participants recruited to the ChatPal trials were given a region specific ‘Trial Code’ so the number of participants in each area could be tracked and recorded. The chatbot sent participants a link to external surveys so they could complete primary and secondary outcome measures, and provide additional feedback on the app. ‘Participant IDs’, which are anonymous identifiers given to each app user, were used to link the chatbot event log data to the survey data for analysis. This meant the outcome data (e.g. measures of mental wellbeing) that was collected in the surveys could be linked to usage data on how those users actually used the chatbot during the trial period (recording via the event log data). This allowed researchers to associate changes in outcomes with the app usage, as outcomes may possibly improve even if the chatbot was not used (for other reasons/confounders).
Advanced analytics beyond simple statistics can be applied to the event log data to gain new insights, such as, applying unsupervised machine learning (clustering) to identify different groups of app users based on usage statistics.
To hear more about the ChatPal trials, follow this link https://www.youtube.com/watch?v=KJc8KoDMi6g - Video where Professor Catrine Kostenius and Frida Lindström (Luleå University of Technology) are joined by Courtney Potts (Ulster University), to discuss the trials that have been undertaken for the ChatPal app.
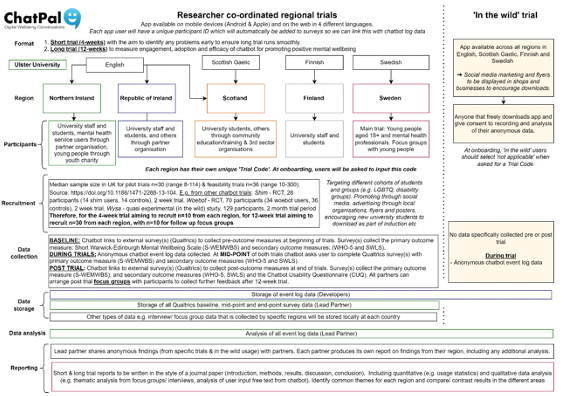
Participants recruited to the ChatPal trials were given a region specific ‘Trial Code’ so we could track the number participating in each area. The chatbot sent the participant links to external surveys to complete primary and secondary outcome measures at various time-points, and provide additional feedback on the app. We used a participant ID (a unique anonymous identifier given to each app user) to link the event log data to the survey data for analysis. Event log data can be analysed to measure uptake and user retention. Advanced analytics beyond simple statistics can be applied to the event log data to gain new insights. For example, applying unsupervised machine learning (clustering) to identify different groups of app users based on usage statistics.
Our recommendations
Plan to test the chatbot in a feasibility, pilot or randomised controlled trial
● Utilise online advertising and traditional marketing approaches to recruit participants, targeting both digital and non-digital natives ⇒
Tips for a successful social media campaign
➔ Allow for at least 3-4 months to build audiences prior to campaign launch
➔ Generally, video campaigns are more engaging than static images, attracting a larger audience
➔ People may engage more with campaigns if reputable organisations/ institutions that have been involved in the design and development process are mentioned in the advertisements (for example, health services and universities) ➔ Ensure the onboarding process is as simple as possible. For example, set up the campaigns in a way which means participants only have to click once to download the app and begin onboarding onto the trial.
● Allow participants to “opt-in” to storage and analysis of their data where possible
● Carry out a short trial to identify and solve any potential problems to ensure the longer main trial runs smoothly
● Consider using multiple scales to cross examine outcomes, or to measure different outcomes
● Use scales that are validated, robust, easy to use, suitable for multiple uses and can be translated
● Analyse event log data to measure uptake
● Report on user retention and how people use the app, and analyse the association between chatbot usage and outcomes Expected output(s): trial plan, marketing campaign to recruit participants, user event log data for analysis, document or report outlining trial results including any changes in outcomes.
Expected output(s): trial plan, marketing campaign to recruit participants, user event log data for analysis, document or report outlining trial results including any changes in outcomes.
Chatbot Service Deployment
The final step is deploying the chatbot service. In terms of the product, improvements can be made to the final chatbot based on feedback from trial participants. It might be worth considering setting up a committee to meet regularly to discuss maintenance of the chatbot. Findings from the trial should be publicised, showing any improvements/ changes in outcome as a result of using the chatbot. An important long term consideration is the end-users trust in digital technology, as this will affect usage and adoption of the chatbot. As with the trials, we recommend a short and concise statement on how end-users data are used, stored and analysed with a link to the privacy policy.
The default option should be ‘opt-out’, so users can choose to opt in if they wish. Guidelines to inform domain experts on how to recommend or prescribe the chatbot helps to provide confidence and competence amongst healthcare professionals, affecting their attitudes towards adopting and prescribing new innovative digital health technologies to clients. See here for example https://chatpal.interreg-npa.eu/general-information-on-chatpal-faqs/. In the UK, the Organisation for the Review of Health and Care Apps (ORCHA) who assess and accredit digital health technologies, published a report on UK attitudes and behaviour titled “The People’s View of Digital Mental Health” https://info.orchahealth.com/digital-for-mental-health-attitudes-and-behaviour-report In this report, ORCHA detailed the results of an online poll of 2,000 UK residents, finding that over a third (36%) of adults would be happy to receive a mental health app recommendation from their doctor. Across age groups this figure rose to 45% for those aged between 35 - 44, and 55% for 18 - 24 year olds.
Thus it is vital to have the backing and support from healthcare providers. Educating and helping staff to accept digital transformation allows them to understand the service so they can engage with clients to recommend trying the app and increase the likelihood of the client using the app longer term.
Branding can influence trust in digital technologies, as people are more likely to trust an app which is endorsed by a health care body, such as the National Health Service (NHS) in the UK, rather than a commercial organisation. For example, ORCHA provide a rigorous assessment process allowing the UK healthcare industry to select quality assured apps. ORCHA assess apps, scoring the app based on data and privacy, professional assurance and usability/ accessibility. If the app reaches a benchmark criteria (scoring 60% or more) it will go on ORCHA’s digital app library so healthcare providers can use their services to recommend apps to clients.
Our recommendations
● Make improvements based on user feedback ahead of releasing the final chatbot service
● Consider how the chatbot can be integrated into current services and how the data flow from the chatbot can inform and enhance these services (e.g. perhaps mood data and usage data from the chatbot can be shared in advance with a therapist to better inform and improve the quality of therapy sessions)
● Set up a long term committee to maintain chatbot
● Take steps to ensure healthcare professionals and clients trust the chatbot service ○ Publicise results of trial showing any changes in outcome as a result of using the service ○ Work with healthcare professionals to clearly explain the service offering so they have trust in the product and can recommend the service to their clients ○ Put app through assessment/ accreditation process so healthcare services can be assured in the technology they recommend ○ Highlight endorsement by healthcare bodies if applicable.
Expected output(s): final healthcare chatbot, evidence base to allow healthcare professionals and clients/ end users to trust in chatbot.
References
- Baumel, A., Faber, F., Kane, J. and Muench, F. (2016) Enlight Quality Assessment Section. Enlight-Quality-Assessment-Section.pdf (mindtools.io)
Bond, R., Moorhead, A., Mulvenna, M., et al. (2019) Exploring temporal behaviour of app users completing ecological momentary assessments using mental health scales and mood logs. Behav Inf Technol 38:1016–1027. doi.org/10.1080/0144929x.2019.1648553
Bickmore, T., Trinh, H., Olafsson, S., et al. (2018) Patient and Consumer Safety Risks When Using Conversational Assistants for Medical Information: An Observational Study of Siri, Alexa, and Google Assistant. J Med Internet Res 20(9):e11510. doi.org/10.2196/11510
Cameron, G., Cameron, D., Megaw, G., et al. (2018a) Best practices for designing chatbots in mental healthcare: a case study on iHelpr. In Proceedings of the 32nd International BCS Human Computer Interaction Conference (HCI ‘18). BCS Learning & Development Ltd., Swindon, GBR, Article 129, 1–5. doi.org/10.14236/ewic/HCI2018.129
Cameron, G., Cameron, D., Megaw, G., et al. (2018b) Back to the future: lessons from knowledge engineering methodologies for chatbot design and development. In Proceedings of the 32nd International BCS Human Computer Interaction Conference (HCI ‘18). BCS Learning & Development Ltd., Swindon, GBR, Article 153, 1–5. doi.org/10.14236/ewic/HCI2018.153
Deibel, D. and Evanhoe, R., 2021. Conversations with Things: UX design for Chat and Voice. Rosenfeld Media. Goldstein, S.P., Evans, B.C., Flack, D. et al. (2017) Return of the JITAI: Applying a Just-in-Time Adaptive Intervention Framework to the Development of m-Health Solutions for Addictive Behaviors. Int J Behav Med 24:673–682. doi.org/10.1007/s12529-016-9627-y
Mori, M., MacDorman, K.F., and Kageki, N. (2012) The Uncanny Valley [From the Field]. IEEE Robot Autom Mag. 19(2):98-100. doi.org/10.1109/MRA.2012.2192811.
Potts, C., Ennis, E., Bond, R.B. et al. (2021) Chatbots to Support Mental Wellbeing of People Living in Rural Areas: Can User Groups Contribute to Co-design?. J Technol Behav Sci 6:652–665. doi.org/10.1007/s41347-021-00222-6
Stoyanov, S., Hides, L., Kavanagh, D. and Wilson, H. (2016) Development and Validation of the User Version of the Mobile Application Rating Scale (uMARS). JMIR mHealth and uHealth 4(2):e72. doi.org/10.2196/mhealth.5849
Sweeney, C., Potts, C., Ennis, E., et al. (2021) Can Chatbots Help Support a Person’s Mental Health? Perceptions and Views from Mental Healthcare Professionals and Experts. ACM Trans Comput Healthc 2(3):1-16. doi.org/10.1145/3453175
Healthcare Chatbot Design Checklist
Stakeholder Centred Design
Healthcare Domain Understanding
- Carry out literature review
- Plan knowledge sharing discussions or workshops between healthcare professionals and technology experts
- Identify use cases endorsed by healthcare professionals
- Determine chatbot scope and purpose
Technological Constraints
- Assess strengths and weaknesses of potential technologies
- Look at open source development frameworks
- Consider opting for a chatbot development environment where data access is private and standalone
- Think about implementing keyword triggers
- Plan chatbot responses to unexpected input/ edge cases Needs Analysis
- Identify relevant stakeholders
- Gather user needs by working with end users and stakeholders (e.g. surveys or workshops)
Defining Requirements
- Co-produce solutions to meet user needs and use cases that professionals endorse, within technology capabilities.
- Prioritise and refine list of requirements
- Establish final chatbot features based on requirements
Dialogue Design
- Finalise design decisions including chatbot modality and use of multimedia or voice, style of interactions, personalisation, and how the chatbot uses external knowledge
- Create conversational scripts based on final chatbot features with conversational design experts and best practices
- Ensure users are aware of chatbot limitations at the beginning of the chatbot conversation
- Think about how user input is stored and represented by the chatbot
- Translate content into other languages (if applicable)
- Assess and sign off scripts based on conversation flow, quality of information, cultural aspects and persona (see full checklist in the toolkit above)
Chatbot Development
- Set up anonymous recording of every interaction within the app (user event log data) for future analysis
- Consider using ecological momentary assessment, to gather valuable user responses ‘in the moment’
- Think about adding multimedia content and global notifications to remind users to engage with the app
Chatbot Testing
- Extensively test chatbot, e.g. using a multidisciplinary team to report issues to development team to fix
- Carry out usability testing with a small group of end users (10-20 participants)
- Utilise user feedback to make improvements to subsequent versions of the app.
Trialling
- Plan to trial the app with end users (e.g. feasibility, pilot or randomised controlled trial)
- Recruit trial participants using traditional marketing (posters, leaflets etc) and via online advertising (see list of ‘tips for a successful social media campaign’ in the toolkit)
- As default, ensure users can ‘opt-in’ to consent to storage and analysis of their data
- Consider a short trial with a small number of participants to identify and solve any potential issues ahead of a longer trial
- Decide on outcome measures and appropriate scale(s) to use at repeated intervals during the trials
- Analyse user event log data from the trials to measure uptake, app users and retention, and explore the association between chatbot usage and outcomes.
Chatbot Service Deployment
- Make improvements based on user feedback ahead of releasing the final chatbot service
- Publish trials findings, showing any changes in outcome as a result of using the chatbot
- Establish how the chatbot can be integrated, inform and enhance current health services by working with healthcare professionals
- Set up a committee to discuss chatbot maintenance long term
- Take steps to ensure healthcare professionals and clients trust the chatbot service (for example, getting services such as ORCHA to review and accredit the app).